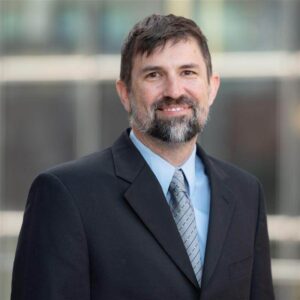
BY Brendan Brown, PWS
CDM Smith
The use of drones, high-resolution satellites, automation, and machine learning are revolutionizing the ability to collect and analyze environmental datasets. These tools are rapidly transforming the scale and efficiency of digital data collection and facilitating the monitoring and assessment of environmental systems to develop comprehensive solutions for the challenges of today.
However, these tools are underutilized for environmental assessment and regulatory compliance. There are numerous benefits to adoption of these technologies to monitor, protect, and restore Florida’s valuable natural resources from seagrass to cypress dome and every ecosystem in between.
Assessing biodiversity and tracking invasive species are vitally important to assessing restoration success, monitoring nature-based solutions, and evaluating wetland mitigation banks. Traditional field methods are labor-intensive, time-consuming, and leave vast knowledge gaps.
Starting in summer 2021 and most recently in summer 2023, CDM Smith collected site-wide high-resolution, multispectral drone data at several west-central Florida wetland and upland restoration sites and then developed custom-built machine learning models to map over 14 common invasive and native species. The model predictions were more than 80 percent accurate and provide land managers with comprehensive site knowledge to drive decisions on invasive species control measures, contractor performance, and future restoration efforts. With these methods, the time from field visit to actionable site knowledge is reduced to a few days rather than several weeks.
In Florida, site development feasibility is greatly dependent on the extent of wetlands versus uplands, due to state wetland protections. Wetland assessments traditionally rely on low-resolution, publicly available data for planning purposes or time-consuming field delineation for permitting.
For a 7,000-acre solar siting feasibility analysis in northeast Florida, CDM Smith developed a custom-built deep learning model to predict wetland extents that integrates plane-acquired lidar (light detection and ranging) and multispectral imagery. These methods provided results based on higher quality data that reduce risk and uncertainty while also expediting the schedule by several weeks. Deep learning models should be evaluated to conduct large-scale wetland delineation for permitting, as it would save months on project schedules while also reducing costly field efforts.
These technological efficiencies allow a shifting of funding and resources from monitoring and assessment to restoration and protection while improving data quality and analysis in support of regulatory compliance and restoration evaluation. Once machine learning models are built and validated for accuracy, they can be applied year over year or month over month to provide objective, timely, cost-efficient, and repeatable analysis of environmental systems.
Regulatory agencies also can benefit from high quality and frequency digital data to drive environmental decisions rather than the limited view provided by traditional field methods and static reporting.
Bringing the promise of digital data and machine learning to fruition will require environmental experts to combine quality field data, remote sensing, and machine learning. We can expedite adoption of these methods by leveraging existing monitoring data to provide model training and accuracy assessments. By developing machine learning models of Florida’s environmental systems now, we can design solutions for today and the future.